Data quality: the lifeline of successful AI adoption
Tags
This article was first published in World Commerce Review
Artificial intelligence (AI) is improving quickly and we are moving rapidly towards a future where technology can complement and augment human capabilities. That means it will soon outperform humans in providing many services, but AI requires large amounts of data to drive the decision-making processes that use machine learning applications.
This is an advantage for the financial services sector which has a long history of accumulating and utilising information. The challenge though is, as the old saying goes ‘garbage in – garbage out’. That is particularly true of machine learning which takes in raw data and converts it into something useful and so needs the right data ‘in’, to get the right insights ‘out’.
Early adopters of AI have applied machine learning to very large data sets where algorithms can detect patterns and learn how to make predictions and recommendations by processing data and experiences. High quality data, however, is vital to the success of this process, and the potential of AI won’t be realised if firms continue to capture data based on out-of-date analogue business processes.
Just being an early adopter is not enough, successful deployment of AI also requires a suitable information infrastructure and a recognition that AI will not fix the loopholes in existing information logistics system. If the infrastructure was originally built to support analogue processes, companies will run into problems, not to mention frustration and lack of trust from leaders when the output is inconsistent. You can build an amazing house, but if your foundation, in this case data, is faulty, it will not matter how well the house was built.
Assuming that the use cases of the AI machine are in place, companies need to decide what data to feed the machine. To define, gather and process that data effectively, they need to set up a governance framework and think about strategies to ensure the completeness and accuracy of the data.
Good data governance is about multi-disciplinary responsibilities
That governance framework is a pre-condition for sound information logistics. The board and senior management can then promote the identification and management of data quality risks and deploy adequate resources to tackle them. It is also important to recognise that the design, build and maintenance of information architecture and the supporting IT-infrastructure is just as important for internal as for external services. To break through organisational silos, responsibility for data ownership and quality assurance needs to be crossfunctional and rest in multi-disciplinary teams.
Complete data means looking in unexpected places
Once a governance structure is in place, an audit of data completeness should take place. To deliver the greatest insights, AI needs data from across a range of functions and multiple sources across the organisation. This needs to include dark data – the hidden data sources that can bring added business value.
Accessing this dark data may mean tapping into the large amounts of information found in excel spread sheets, or unstructured data such as communications (for example phone, mail, chat or the digitalisation of documents that have been archived). To mitigate the risk of human errors, data aggregation should, where possible, be automated, making it important to check the level of automation of processes, especially repetitive ones involving substantial amounts of data.
Accuracy by engineering the single point of truth
The ability to generate accurate and reliable data that supports internal and external services is the core ambition for most businesses. But without a unified process and sound metrics there will be a gap in data orientation, making it difficult to detect which data is ‘white noise’ and which is genuinely valuable. Inconsistent labelling due to a lack of data models and standards between systems in the company is usually the cause of this confusion.
Another problem is that business rules might have been implemented inconsistently, making it difficult to understand how data is changed while processed. The result can be an unnecessarily complicated architecture, often referred to as ‘hairball architecture’. The opposite, and most desirable, option is to create a centralised information architecture where standardised data is collected and entered only once to provide a single version of the truth.
The cost of erroneous data in decision-making can be extremely high and employing departments to analyse information and make corrections is costly and time consuming. It also means organisations can become much slower to change, for example in response to competition, service innovation or new regulations. Implementing a principle of having one version of the truth simplifies the management of the data and keeps quality consistent over time.
There is no shortcut to high quality data. Hard work and focused investments are needed to get to the root cause of any issues. This is often forgotten when AI projects are initiated but as the project develops, companies will face escalating budget demands to fix data issues lower in the pipeline. As in building a house - it’s better to start with the architectural plans, rather than the interior design of the penthouse.
No substitute for becoming totally digital
It is not possible to leapfrog a fundamental digitalisation journey and go straight to AI-enabled decision-making. The scale of investment in technology and people required can be huge, but a fundamental digitalisation transformation is needed before AI decision making can be enabled. As the industry is facing an increasingly competitive environment with pressures on margins, downstream cleaning and cleansing of data will not be economically viable.
AI is just one of many new technologies that can deliver more value to external and internal customers, but they will only create value if the data that feeds them meets their needs. Firms cannot prepare for AI by looking at the symptoms of data problems, they need to address the root causes of any poor data quality.
So in summary, there are three critical steps needed to build a robust framework that enables true digitalisation and AI applications. The first is to build a cross disciplinary data governance framework that has the authority and budget to implement change. The second is to carry out an audit of data completeness throughout the organisation to understand what and where data is, including dark and unstructured data, and promote automation for data aggregation.
Finally, organisations need to move toward a centralised system architecture where the goal is that standardised data is collected and entered only once. These steps may be costly and they will take time. But if these fundamental changes are not made, organisations will just be putting band aids on a broken system.
Explore more
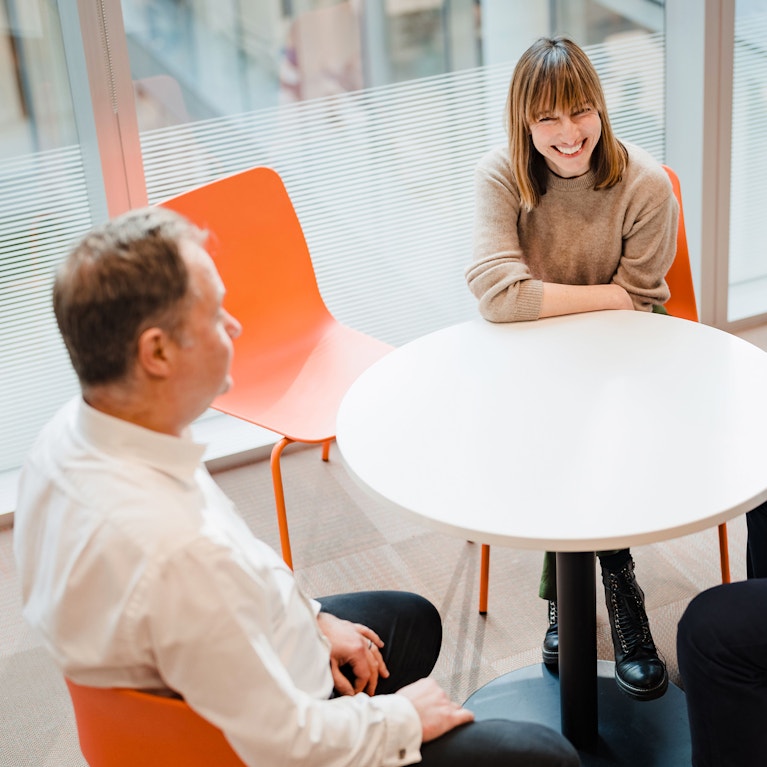