Using data analytics to improve project management in the nuclear industry: Life beyond the dashboard?
Tags
This article was first published in NIA Industry Link Magazine
The nuclear industry is poised to play a significant part in the UK’s journey to net zero but is hampered by historic underperformance in delivery, and consequently has a poor reputation. As opportunities for new projects emerge, both in decommissioning and new build, building trust in the sector to deliver will be critical to securing the public and private funding required to make these projects happen. But the nuclear industry has a consistency problem when it comes to delivering projects and programs – and we don’t seem to be getting any better. One factor that could change this is that digital transformation within the engineering, construction and project management sectors is increasing the amount and quality of tools, techniques, data and delivery approaches available – and these are creating entirely new capabilities.
We’re struggling to get better at delivering projects and programs
While there are lots of examples of good practice and performance in project and program delivery in the nuclear sector, unfortunately some of the highest profile projects/programs have not performed well, and some have even been abandoned – which could reflect a lack of confidence in the sector’s delivery track record.
The reality is that despite the advances in project/program management methodologies (enabled by digital transformation), we don’t seem to be improving at delivering these kinds of complex major programs. In 2021 the UK Government’s Major Projects Portfolio comprised 184 projects totalling £542Bn in whole life costs. According to the UK Government’s Infrastructure & Projects Authority (IPA), nearly four fifths of these have significant issues or are unlikely to deliver successfully. Previous years’ data show similar results – indicating a lack of progress.
In addition, analysis by Oxford University’s Said Business School concluded that for all nuclear power projects contained within their database, there were cost overruns in 100% of projects, while schedule overruns occurred in 90% of projects[1]. Whilst this database isn’t UK specific, and doesn’t capture every project the industry delivers, this is still a sobering statistic.
What aren’t we improving more quickly?
Nuclear industry projects and programs are becoming more and more challenging as the external environment in which they are delivered becomes volatile, uncertain, complex and ambiguous (VUCA – a term coined by the US military). Complex programs typically have dense interdependencies and exhibit complex system properties. This means that when one element changes (scope for instance) this can lead to non-linear change propagating throughout the ‘system’. For program leaders, this can quickly lead to programs becoming unpredictable and extremely challenging to manage. It is therefore becoming increasingly difficult to make well informed, timely decisions and achieve successful outcomes, and this is impacting organisations’ revenue, return-on-investment and reputation.
Is data analytics the answer?
Data analytics can have a significant impact on improving decision-making. PA Consulting’s innovative flexible modelling and analytics solutions have helped inform critical ministerial decisions, strategic program delivery and target setting for the UK vaccination program, directly driving the administration of more than 9 million vaccines in the first two months of the program.
In the nuclear industry, there is unexploited value in the data generated and held by projects, but also within the systems of interactions connecting the building blocks of major programs. Unlocking this value will enable leaders to make better decisions, and improve the performance of their projects, program and portfolios, and ultimately, maximise return on investment. Whilst we see the primary benefit of data analytics as helping nuclear project and programme leaders make better decisions, there are other potential benefits too:
- Free up PMO teams’ time to do other more valuable work by speeding up or automating analytical tasks
- Improve morale by automating monotonous repeatable tasks
- Unlock entirely new capabilities not previously available by blending different technologies and analytics techniques (e.g. monitoring construction progress intelligently and automatically using camera footage from drones)
- Reduce intervention times on emerging risks or issues, reducing their cost or schedule impact by speeding up data acquisition and interpretation
- Reduce waste due to re-work by predicting areas of scope where this is more likely
The idea of using data analytics to support the management of projects and programs is not new, nor is the knowledge and understanding of data science and systems thinking techniques. However, it is worth defining what we mean by data analytics when applied to project management. Data analytics is systematic analysis of data or statistics used for the discovery, interpretation, and communication of meaningful patterns or insights to aid effective decision-making, and is often confused with artificial intelligence. The two are different – artificial intelligence is an umbrella term for wide-ranging computer science techniques to build smart machines capable of performing tasks that typically require human intelligence.
Life beyond the dashboard?
The dashboard is now ubiquitous amongst project and program teams for visualising management information. Dashboards add value by distilling and visualising key data in a meaningful way so that it can be used by decision makers to course correct or respond to emerging issues (this is known as descriptive analytics). However, most dashboards have a key limitation in that their datasets are a view of the past, providing only hindsight. Some organisations have driven further value from data exploring interdependencies and their effects on performance in ways not previously done (yielding insight). However, in most nuclear industry organisations, dashboards and the data processes that sit behind them currently do little to provide foresight.
There will always be a need for management information, including historical data, and dashboards (or visual reports) are an excellent medium to display these – especially if they are intuitively designed. However, we must look beyond dashboards that contain solely linear historical data if we are to truly realise the potential of data analytics in the nuclear sector.
Achieving this is not necessarily about gathering more sources of data (although we believe there are unexploited sources available, which we explain below), but rather extracting greater insight, or even foresight, from the sources we have (or could have) access to. For instance, historical performance data does not tell us what is happening within a project, it just tells us how the activities of the project within its environment have manifested themselves in a range of pre-defined metrics (such as Earned Value Metrics CPI and SPI). We argue that this does little to help a decision-maker to make corrective action, because the ‘why’ is missing. Similarly, historical data shows a trend, which can be (and often is) extrapolated into the future. But historical data can be mistaken for future certainty, and as today’s world is more ‘VUCA’ than yesterday’s, we must find new ways of understanding and dealing with that uncertainty.
Below we outline key data analytics and artificial intelligence techniques including – but also beyond – dashboards (descriptive analytics), and their potential use cases in projects and programs in the nuclear sector.
Descriptive analytics involves collating and presenting data to enhance its meaning, often using data visualisation techniques/software. Example use cases include project performance and progress reporting (e.g. dashboards using PowerBI), analysis of drivers and root-causes of past performance. For example, we helped the Foreign and Commonwealth Office drive prosperity and combat global poverty more effectively by building a cloud-based system called Prospero to monitor and report on the performance of programs across its £1.2Bn Prosperity Fund. Its analytics let civil servants, ministers, diplomats and other officials track exactly where money is spent and the results of spending that money.
Predictive analytics involves using historical data combined with modelling to predict future performance. This can deploy machine learning, which uses models that iteratively learn from data and can find hidden insights without being told where to look. Use cases relevant to nuclear sector projects and programmes include modelling of multiple future performance scenarios to ascertain risk or uncertainty or machine learning applied to historical project data (time, cost, benefit) to forecast future performance.
Prescriptive analytics involves solving to identify a preferred solution or course of activity based on a set of defined input parameters, and can include neural networks, complex event processing and machine learning. Use cases relevant to nuclear sector projects and programs include developing smart recommendation engines for advantageous purchasing of commodities (such as steel) as market prices fluctuate, or identifying optimum combinations/sequencing of activities based on strategic priorities or constraints. For example, we helped a utility company think about everything at the same time when considering their asset maintenance portfolio. We built a portfolio optimisation engine that delivered multi £m annual cost savings and improved the return on investment from multi-year investment decisions by solving for optimum portfolio composition and timing, using a multivariate constraints and parameter input framework for each portfolio.
Computer vision involves the automatic extraction and analysis of digital images and videos, enabling for example significant automation opportunities in complex environments. Use cases relevant to nuclear sector projects and programs include digitising legacy engineering documentation (e.g. scans of power station as-built drawings) to embed in new digital workflow and document management. For example, we helped a large engineering asset operator develop a high performing automated digitisation of thousands of paper engineering drawings, and save 26 weeks’ work of expert time each year.
Natural language processing (NLP) is used to “make sense” of semi- or unstructured natural language data – textual and auditory. Most NLP techniques rely on machine learning to derive meaning from human languages. Use cases relevant to nuclear sector projects and programs include automatically scanning project baseline documentation following a change control, to rapidly ascertain the impact of a contractual change. For example, we developed and delivered a proof of concept enabling automated review of nuclear safety case documentation for a UK decommissioning organisation, which reduced the time needed to review a single document from hours to seconds.
Robotic process automation (RPA) is a technique that enables the automation of digital workflows using a combination of user interface technologies. Use cases relevant to nuclear sector projects and programs include automatically generating project progress reports that extract data from a variety of software.
BIM, GIS and Digital Twins use geospatial, geometric or physical performance data to define or model physical assets or environments. Use cases relevant to nuclear sector projects and programs include optimising construction or logistics sequencing using digital twins of whole sites or using GIS data captured from drones or satellites to monitor progress or weather risks. For example, we built a digital twin to optimise the maintenance of a steam distribution sub-system for a nuclear operator, identifying £2m savings per year on the sub-system alone (around 3% of the overall system).
Overcoming barriers to innovation and adoption of data analytics in nuclear projects
Apart from a few leading edge organisations and technology startups, scaled adoption of these disruptive techniques is yet to happen. That slow pace of adoption indicates that this isn’t a data science problem, the technology, methods and knowledge are available, rather it is an innovation problem. It is clear that there are a number of barriers to innovation and adoption including problems with data sharing, a lack of incentives and limited knowledge and focus on what is available. Below we expand on these, and explain how these could be overcome in the nuclear sector to realise the value of data analytics to drive decision making.
Fragmented and unexploited data – think big, start small
Data is held on different platforms, often within different organisations, and the variety of project management software used creates silos that often require manual intervention when amalgamating data. With any emerging field, the number of software developers will only increase, further fragmenting the market creating a need to develop ways to work with varying data sources. Building PMO teams with skills in coding could become the norm in future to address these challenges.
In addition, commercial sensitivities in current contracts make it hard to share data. Developing novel commercial constructs that unlock the sharing of data across organisational boundaries will be key. Whilst the idea of data trusts is being discussed, we are a long way from this becoming the norm in nuclear sector commercial agreements.
The other side to this is data that is available but we are not currently exploiting. Projects and programs are mature in their capture and analysis of cost, schedule and risk data. There are emerging technologies that are trying to make use of real world data (e.g. drones to support decommissioning project planning), but also other sources such as public sentiment which could be harvested using techniques such as data mining and sentiment analysis.
The way to overcome these barriers is to think big but start small. Leaders should create an ambitious vision, but start with a pilot or number of pilots and don’t be afraid to fail. Decrease the time to value by delivering benefits as quickly as possible to maintain momentum, these are change initiatives after all.
Innovation is hard, and it’s not incentivised in a project environment – change needs to be led from the top
Developing and adopting disruptive technologies is challenging (the Technology Readiness Level valley of death is a well-known phenomenon), especially in highly regulated industries such as the nuclear sector. In addition, project and program leaders are measured on their ability to drive towards certainty – of cost, schedule and benefit outcome. Innovation (trying and testing new technologies that could improve project delivery) is inherently risky, and so why would a project manager want to try something new and risk not achieving their objectives, when they are comfortable they will achieve them without the new technique?
To overcome this barrier requires support from the top. Innovation is uncertain and some initiatives will fail whilst others succeed. There will be a cost to change, and leaders need to seek support from senior management to give them the freedom to experiment.
Lack of understanding of (or envisioning) the art of the possible – acquire the right expertise
Data science and use of advanced analytics is a relatively new field (in the context of the evolution of science and mathematics) and not a mature capability within most traditional project delivery organisations. The pace of change in technologies such as cloud storage has enabled storage and access to large volumes of data – but project and program leaders and organisations are struggling to explore the potential. This is largely due to a lack of knowledge, but also partly because organisations haven’t invested the time in creating a vision for how they will manage projects in the future.
To overcome this barrier, organisations should bring in the knowledge to help them develop the art of the possible, based on their unique organisational context. This may be inorganic to begin with (e.g. experts or consultants) but eventually should shift to organically growing the capabilities of the future (e.g. coding and data science expertise within the PMO).
[1] Research from B. Flyvbjerg and A. Budzier, Said Business School, Oxford University
Explore more
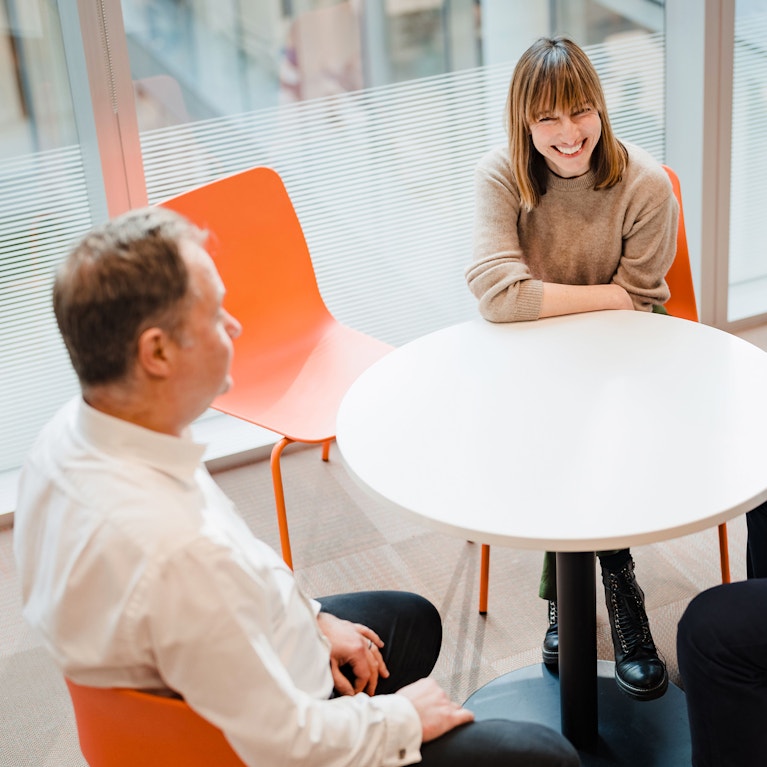