A framework for trust in AI
Tags
New model for Algorithm Assurance checks whether AI is doing what it is supposed to do
Artificial Intelligence is increasingly influencing our decision-making. Checking the operation of the intelligent algorithms that interpret our data is essential to maintain confidence in these solutions. Classic assurance models no longer appear to be sufficient.
The amounts of data that organisations produce and collect are increasing exponentially. More and more organisations are doing their best to unlock the hidden treasures in that data. Data analysis provides valuable insights that enable companies to serve their customers better, make more accurate predictions, better manage risk, reduce the risk of fraud and discover patterns that feed into new business models.
The analysis of large amounts of data has long ceased to be manual work. Organisations rely on automation for advanced data analysis and algorithms have taken over that analysis. Artificial Intelligence and Machine Learning help to continuously improve analytics and tailor insights more effectively to generate the greatest possible value for the business.
Such "intelligent" algorithms are increasingly being used on the ground in production environments, where their insights and findings can have direct consequences for customers, employees, suppliers and the business as a whole. As the potential impact of those algorithms grows, it also becomes more important to develop good methods to control and monitor their operation.
Organisations want to make sure that automated operational processes adhere to all their policies and continue to deliver the intended results. And customers, users and other parties who may be dependent on the conclusions, want to be able to trust that the algorithms used are unbiased, safe and that they comply with all laws and regulations.
The existing assurance approach is inadequate
IT assurance programmes have existed for years and been used to give people and organisations confidence in their IT. Unfortunately, the methods used for such assurance do not appear to be suitable for evaluating algorithms. First, this relates to the algorithms themselves, which are often extremely complex. They often contain mechanisms that cannot be captured in logical "business rules", making these mechanisms difficult for the human brain to imitate.
In addition, the circumstances under which the current algorithms were created present challenges.The promise of data analysis is great so there is a lot of pressure on organisations to unlock the potential value quickly. The focus is therefore mainly on speed of innovation, at the expense of oversight and it is often unclear exactly who in the organisation is responsible for an algorithm.
For example, there is a risk that organisations will start using algorithms before all mechanisms to check they are operating correctly have been fully set up. A design for assurance approach, in which control mechanisms are included in the algorithms in the design phase, is not yet standard practice. This may mean, among other things, that auditors have to try to analyse afterwards how the algorithms work exactly ("post-assurance"). Existing assurance frameworks are generally not designed for this.
Finally, providing assurance for algorithms becomes even more complicated because ethical questions come into play. This is not only about well-known factors such as security and privacy, but also, for example, about the question of how algorithms deal with possible bias, contamination or manipulation of the datasets. In most existing algorithm assurance frameworks, these aspects are indeed considered, but they generally provide basic recommendations, without specifying what must be done in practice to solve the problem.
Algorithm Assurance and AI Assurance Support
As algorithms increasingly influence important decisions that can directly impact organisations, people and society, it is essential to maintain trust in AI models. Rabobank and PA Consulting have therefore jointly developed a new and robust framework for Algorithm Assurance, which (in contrast to many existing frameworks) takes account of the full life cycle of AI models. This enables auditors not only to identify sensitivities, but also to indicate in concrete terms what needs to be changed in processes, organisation or technology, for example, to solve the problem. The framework has now been applied to algorithm-related assurance cases, and, as a result has already proved its worth in practice.
An essential part of this Algorithm Assurance approach is a new risk management framework. With this AI Risk Control Framework, the risks associated with the use of AI models are carefully and fully mapped out. The framework is based on the life cycle of algorithms from initiation and development to production, to eventual discontinuation and the correct attachment of the models and data used. The robustness of the algorithm is addressed, but also the organisation and governance around it, the requirements for use in the production environment, and data management. In addition, specific attention is paid to "trust" risks, such as data privacy, security and ethics. The framework is a growth model that takes into account new insights in the field that can be incorporated directly into it.
In addition, at the end, where the output of the AI models has to be tested, a new solution has been developed (AI Assurance Support Model), which is able to test the results of AI models, even if the underlying algorithm itself is not accessible (a 'black box' approach, as can be the case with SaaS solutions, for example). This support model, in turn, uses AI to control the inbound and outbound data flows and for the logic of the AI model to be tested. Rabobank and PA have drawn three main conclusions about these new Algorithm Assurance solutions when compared with conventional (IT) audit approaches.
Firstly, it appears to be essential to bring the worlds of assurance, IT and data science together. For example, an experienced data scientist can ask the right questions about the AI model, so that a good picture is created of how it was designed and built and why certain choices were made. A high level of data science experience is also required to develop the AI Assurance Support models. A joint approach and process is a prerequisite for achieving reliable Algorithm Assurance.
Second, experience with the AI Risk Control Framework and AI Assurance Support clearly shows that the largely linear approach of conventional audits is inadequate. A statistically relevant Assurance Support model can only be developed in an iterative process that must be carefully monitored by the auditor.
The third and most important difference from the conventional approach is that Algorithm Assurance actually shows whether AI models really do what they are supposed to do. This addresses concerns and distrust from management, employees, customers and regulators. Thanks to the application developed by Rabobank and PA, using Algorithm Assurance in practice provides positive assurance that algorithms using artificial intelligence actually do what they are supposed to do.
Explore more
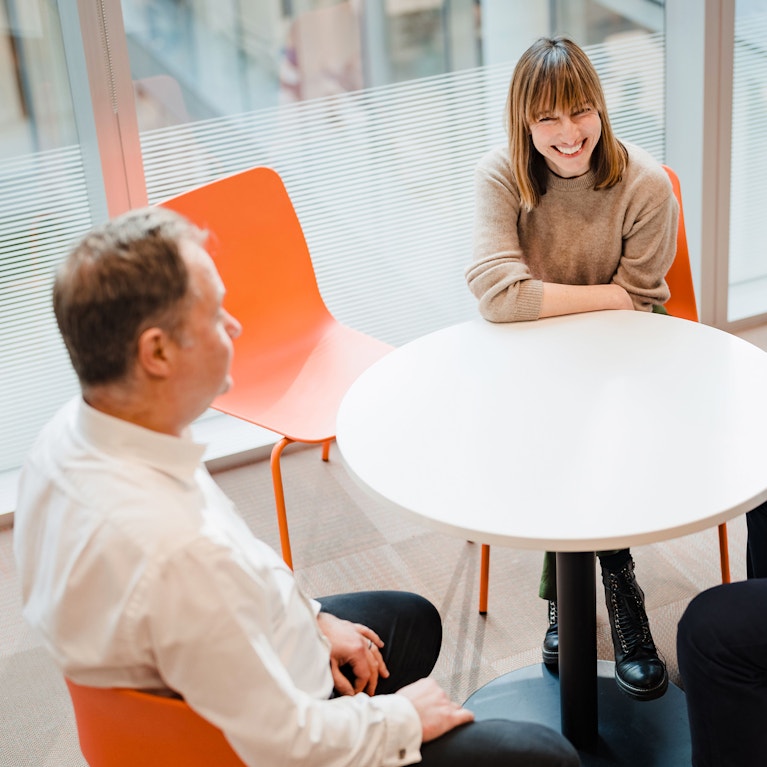