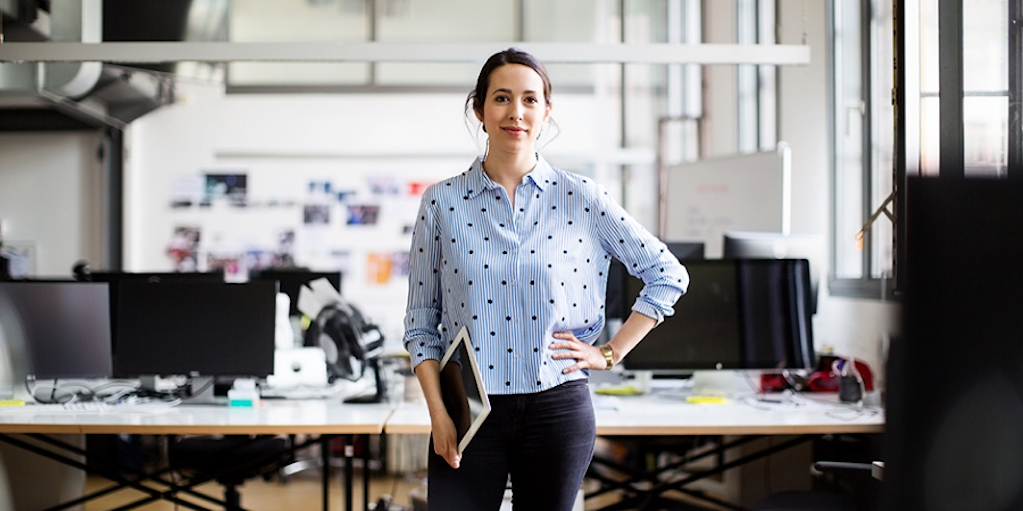
Privacy in the automation age: How to manage privacy risks when adopting artificial intelligence and machine learning
Artificial intelligence (AI) and machine learning (ML) are creating more privacy risks as they become a common business tool.
In recent years, they’ve gone from futuristic experiments requiring the budgets of large companies, to commodity cloud offerings that are readily available to anyone.
While the ability of AI and ML to take over laborious tasks and rapidly generate insights can be great for productivity and innovation, they also come with risks – as there’s little regulation of these data-driven tools, it’s easy to underestimate their power and fall foul of unexpected consequences. For example, AI and ML can inadvertently expose private information by linking disparate data sources with greater ease and at a larger scale than ever before.
Such risks can make people nervous, opening the door to challenge from privacy groups. And when things do go wrong, the negative publicity can cause significant reputational damage.
Skilled governance is key to minimising AI privacy risks
To avoid the privacy pitfalls of adopting AI and ML, private organisations can learn from the strict legal frameworks that government departments use. They can create a skilled governance board to oversee the implementation of three key principles: necessity, proportionality and authorisation.
The governance board should include representatives from the legal and policy community, as well as ones from the team managing the AI or ML system and customer community. This diverse expertise lets the governance board have open discussions about how the organisation wants to use data to power AI and ML, and the benefits it expects to gain.
By considering these questions in terms of the three principles below, the governance board will be able to understand how the use of AI and ML will impact privacy.
1. Necessity
The organisation should be able to demonstrate ‘why’ a particular data set is necessary, as well as the insights and benefits they expect to gain from using the data.
Many organisations argue that it’s too difficult to know what insights they might gain before onboarding and interpreting a data set. However, this argument only shows that the organisation is unclear about what it’s trying to achieve through AI and ML.
If you can’t define your goals, you should be wary of unleashing the full power of AI and ML on your data.
2. Proportionality
The organisation should be able to show they’re using the data set in a proportionate way – that is, they’re not invading people’s privacy or simply adding the data set into the system as it ‘might be useful’.
It’s also important to consider how the system could interpret the data when combined with other information. For example, an organisation might use a summarised data set, such as counts of people rather than data sets directly related to individuals, to protect privacy. But the AI or ML system might expose individuals when cross referencing with other data sets.
3. Authorisation
Organisations need to be clear on how they’re using data sets and confirm that this is within the terms of use, including whether there are limits on processing the data. There’s a lot of open-source data available to organisations, but it usually comes with terms and conditions.
Educate customers about AI privacy
While following these three principles will help avoid privacy issues, it’s also still important to educate customers to allay concerns about the use of their data and the application of AI. So, organisations should be open about how they use data, apply AI and ML, and protect privacy. The UK’s GCHQ, for example, has issued a paper about its plans to adopt AI over the coming years.
Organisations that have skilled governance to ensure their use of artificial intelligence and machine learning is necessary, proportionate and authorised, and maintain an openness about it, will be able to make the most of the technology while maintaining privacy.
Explore more
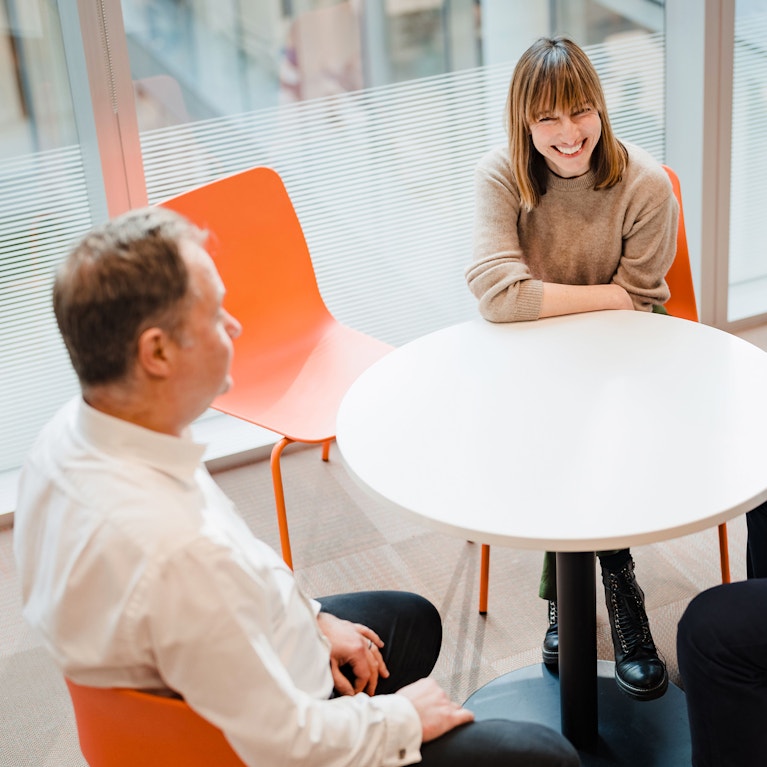