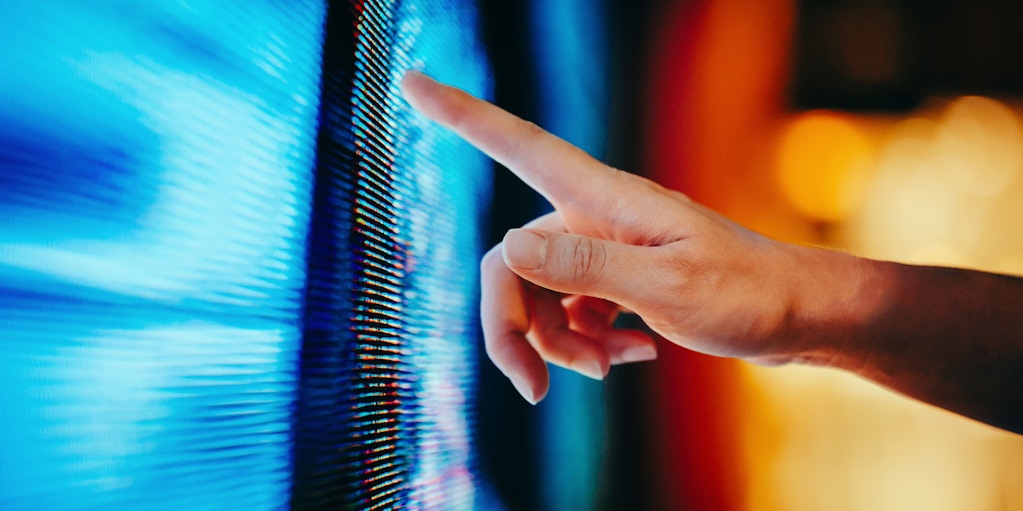
Harnessing the power of AI to accelerate the energy transition
Tags
ChatGPT is one of the most advanced chatbots ever created. Launched in November 2022, it had one million users in its first week alone – you might be one of those who’ve already tested its generative writing skills.
Generative AI tools like this are the result of decades of advancements in AI. Significant progress in the field of Natural Language Processing (NLP) technologies have led to language models that have progressed from text mining to natural language understanding and engaging in human-like conversations. This capability has arrived at a crucial time and could unlock some of the most complex challenges that energy and utilities companies face right now - including, but not limited to, meeting net zero goals.
Whilst the relentless advancements in AI and the proliferation of new tools offer near limitless potential, there has been a lot of nervousness across the industry. Traditionally, we face our fears and anxieties gradually, by progressively developing the skills and resilience to navigate difficult situations and build confidence at the same time. Similarly with AI, organisations must build their understanding of how generative AI can impact their business, as well as their confidence in adopting emerging technologies by building an AI studio that turns vision into reality - from opportunity identification to building the infrastructure and deploying new AI solutions.
AI has an integral part to play in helping energy and utilities companies achieve Net Zero
Many organisations tend to associate the term “data” with quantitative information. However, the majority of data within organisations is text information locked away in either paper or digital reports. For example, these can include regulations or legislation that you must comply with, codes of conducts or company processes to follow.
Deploying game-changing AI solutions will enable energy and utilities companies accelerate specialised manual processes from months to minutes. Cutting through the complexity of existing data siloes and solve new challenges that would otherwise be impossible.
Below are three examples of why AI has an integral part to play in helping us achieve Net Zero:
- Tackling net zero and ambitious Environmental, Social and Governance (ESG) goals – Monitoring and measuring progress is vital to understand how things are going and where improvements could be made. Advanced AI solutions could automatically and routinely evaluate how well organisations are performing by cutting through the noise in ESG reports and seemingly non-standardised metrics.
- Building our first industry-wide nuclear LLM is critical to the nuclear industry’s knowledge management – Leveraging LLMs will significantly reduce rising operational costs in decommissioning, by helping plug the knowledge gap that will be introduced as senior engineers retire in the next decade, and to understand what the “value” of our data is. Similarly to Sellafield Ltd’s recent AI advancements, it is key for nuclear organisations and other highly regulated and complex organisations to start shaping their AI strategies as well as building AI solutions tools, like SLComply.ai, that can accelerate manual tasks from months to minutes. The safety case of the future does not need to be limited to Microsoft word.
- Build design drawings instantaneously using text prompts will help accelerate future energy construction programmes – Generative AI is already disrupting many gaming industries with tools flooding the market that can build 3D graphics and entire video games through a set of text prompts. Engineers should start to look at how these tools. could be used for technical drawings, introducing game-changing operational benefits and the ability to update designs immediately.
Too good to be true?
Whilst the list of opportunities for AI seem to be limited only by our imagination, it is important to be aware about its potential limitations.
The technology landscape within energy and utilities can be extremely varied, with some players further ahead than others. Highly regulated industries tend to be slow to embrace new technology, while consumer and manufacturing companies have been early adopters of AI models to drive a better understanding of their customers’ needs. These differences aren’t just about the technology, but also about the mindset of different parts of the industry.
- Bias in training data – this is one of the most important considerations when training / tuning an AI model. In addition, is important to introduce safeguards around AI ethics to make sure that this technology is used appropriately.
- Getting the right people involved is key to building confidence in these technologies – whilst there is a growing pool of data science and AI practitioners, there’s a shortage of experts who can evaluate the impact Generative AI can have on an organisation and raise this to board level discussions. This drives the right transformations rather than experimenting with siloed proof of concepts.
- Data maturity – models that rely on historical data may require a significant level of transformation or processing to start training Generative AI LLMs. AI can "hallucinate" and make up versions of its own reality if the datasets whilst training/tuning models are not complete or accurate.
Complex change calls for an AI first mindset
AI isn’t a magic bullet. Not everything is necessarily an AI problem, however, it is important to start adopting an AI first mindset, as this allows us to spot opportunities to innovate as well as solving our biggest pain points.
AI needs to become a board and CEO-level discussion
FactSet searched AI mentions in transcripts of earnings conference calls of all the S&P 500 companies from March 15 through May 25. Whilst 110 companies discussed this technology, none appeared in the Energy or Utilities space.
In the last decade, most data professionals have focused on being “data driven” to enable organisations to make near real time data-driven decisions. The trap is to think that the next step is to be simply “AI-driven”. At a fundamental level, data, digital or AI driven transformations need to be first and foremost “value-driven”. Whether this is achieved through better data or adopting LLMs is secondary.
The energy transition is a highly complex environment. Whilst no one knows which technology organisation will win the race and what the uptake and acceptance of these technologies will be, what is certain is that they all have an important part to play in helping us achieve Net Zero.
Where to start?
Align your AI journey to a simple mission statement
When starting with AI, it is important to build around a simple mission statement that everyone at all levels can relate to. For example, when creating the vaccines technology and data infrastructure, the mission was simple – support the delivery of vaccinations, and the metric even simpler – number of vaccinations per day.
When selecting your first AI pilot, always tie it back to your mission statement as this will make it easier to win hearts and minds at the beginning which is key, and reduce the risk of your pilot becoming yet another proof-of-concept.
Concept evaluation
Whilst focus should always be on accelerating the path to value, leaders often skip the concept evaluation phase. This is a mistake.
This first phase is about framing the problem statement and as a rule it should always be a very short phase (3 weeks max). It allows you to look around within your sphere of influence and fix the things that announce themselves as in need of repair.
The key is not to jump on the first use case, but instead map and prioritise potential AI Proof of Values using first a high-impact – high-feasibility lens to create an initial shortlist. The best AI solution candidate is found by subsequently overlaying each shortlisted use case against its technical building blocks. As a result, adopting this method ensures you can drastically accelerate the development of future solutions that are underpinned by the same AI techniques.
Build the AI Studio
Tackling AI builds for the first time, especially Generative AI, will mean new skills are required. This is not an extension of your IT function, but instead this is a multi-disciplinary team that bridges business, data and emerging technologies to translate idea into reality in weeks and months not years.
Each AI studio needs to have a clear remit, accountability and dedicated leadership, as its role as your execution muscle is to develop AI solutions with transparency and trust, as well as build a future AI capability.
Prove the value
This is the most exciting phase, where the AI studio designs, develops and deploys its AI solution. Whilst the design can range from leveraging existing products to fully customised and tailored algorithms built from scratch, there are two parameters that remain constant:
- Every solution needs a user-centric design to ensure user adoption at the end.
- The AI studio should never stop talking to the business / its end-customer.
One of the key differentiators an AI studio brings is its ability to relentlessly iterate from the continuous feedback it gets from both its design and development.
Finally, once the solution is deployed, the team will drive adoption of its solution by training its future users, which will only be possible if the solution was designed with the end user in mind.
Today, the barrier to entry is the lowest it’s ever been in this space which means all of us have access to very sophisticated algorithms. Therefore, whilst there are significant large-scale investments into the AI market, it is important not to confuse this with cost of development. There are many established techniques and pre-trained models that can be leveraged as-is and that can help automate manual and specialised processes with minimal computational cost.
Scale and continuously iterate
This is the most important phase. It involves upgrading your solution beyond its test user group and embedding it across the entire organisation. The AI studio’s composition shifts to introduce the skills required to productionise and embed the change, providing both the dedicated training to its future users, resolving live issues and introducing new features based on feedback.
However, there are countless proof-of-concepts or values that have successfully demonstrated the use of ground-breaking technology to achieve game-changing benefits but who unfortunately stay stuck at the pilot stage.
Often, this is a result of two important challenges:
- Lack of capability to maintain and support the embedding of these solutions.
- Disconnect between the business and IT functions, which itself can be a result of the mission statement not having been driven top down from the organisation’s leadership.
While generative AI tools will no doubt act as a catalyst in energy transition, without a sustained focus from the leadership, organisations risk being left behind as an AI-led transformation takes place across the industry.
If you want to understand the impact Generative AI will have on your organisation, or if you are ready to take that first step in applying this technology, contact one of our experts.
Explore more
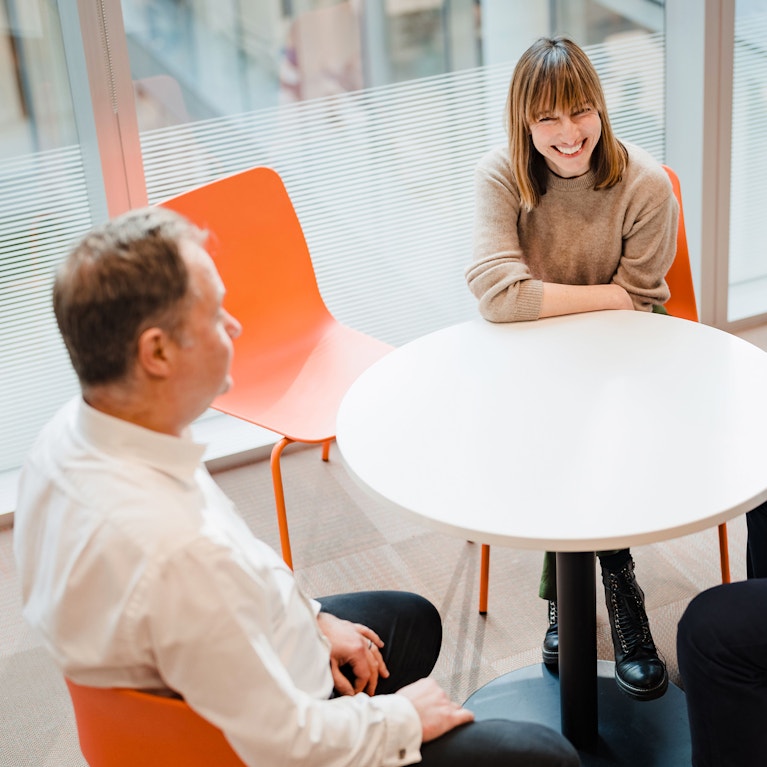